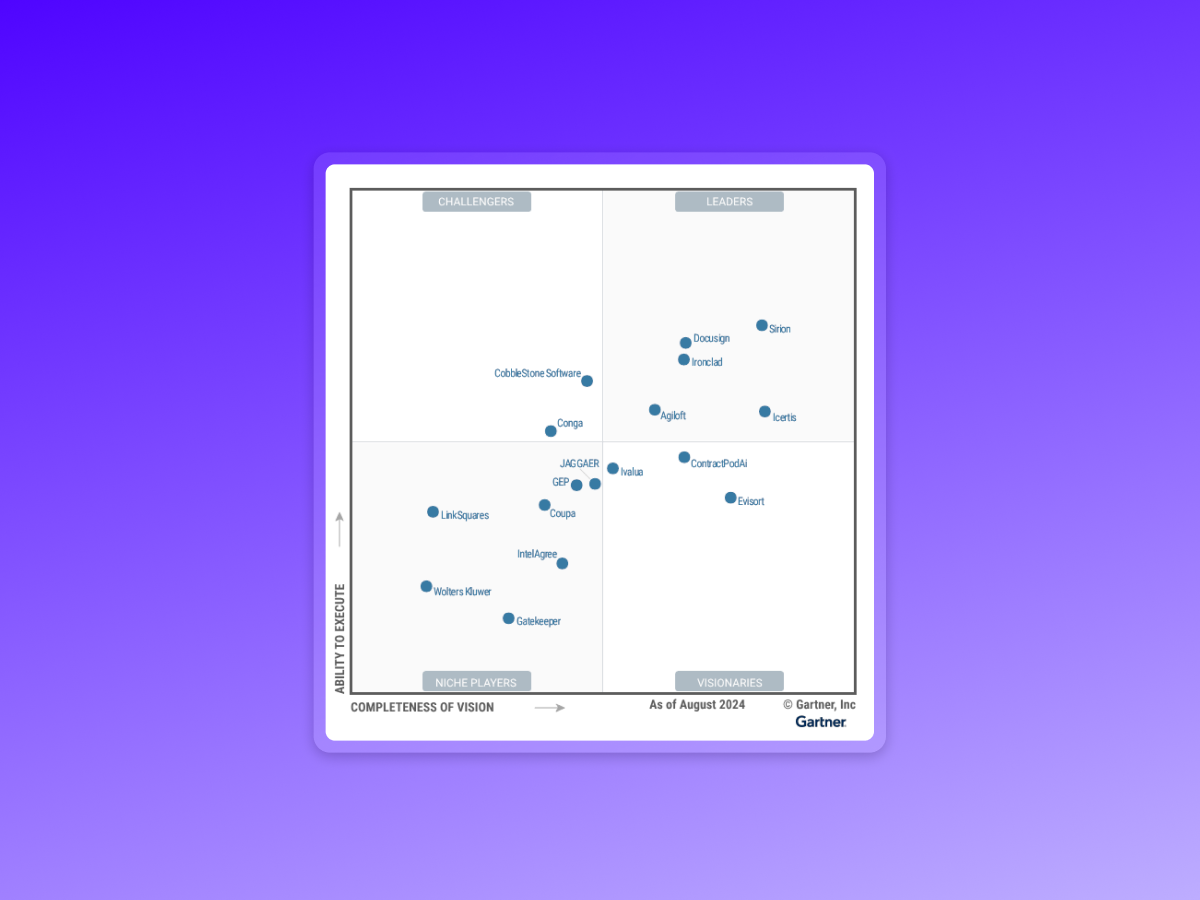
The Security Implications of ChatGPT and Other AI Technologies
Business leaders and security professionals need to be aware of the security implications of generative AI technologies, such as identity theft and fraud.
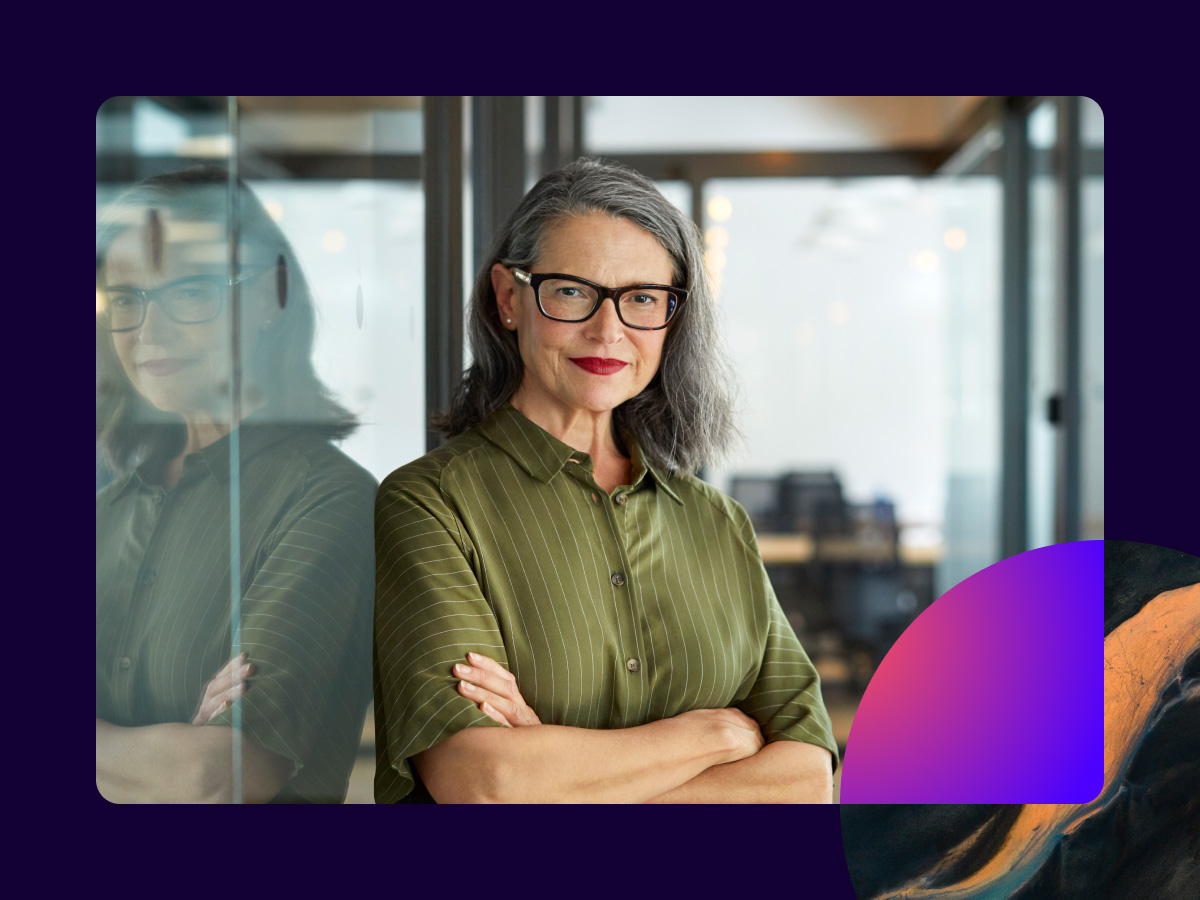
The public release of ChatGPT sparked an explosion of interest and experimentation with the latest generation of generative artificial intelligence (GAI) tools. As awareness grows of the truly vast universe of potential GAI use cases, we’ve also seen a wide range of concerns from fraud and intellectual property theft to out-of-control autonomous war machines.
In this blog, we’ll focus on two areas that business leaders and security professionals need to be aware of:
Identity theft and fraud by cybercriminals using AI to fool identity verification systems and aid social engineering—as demonstrated in a recent 60 Minutes report
Security risks created by employee use of AI tools, such as a series of incidents at Samsung which helped prompt advisories and bans by companies including Apple, Amazon, Verizon, and JPMorgan Chase
Preventing AI-enabled identity theft and fraud
Artificial intelligence tools already present a clear and present danger for identity theft. Able to imitate specific human voices, mannerisms and even speech patterns, GAI tools can be used to fool traditional identity verification methods such as live video and voice calls. The European Union Agency for Cybersecurity (ENISA) has warned organizations of possible face presentation attacks in which photos, videos, masks and deepfakes are used to impersonate users for identity verification purposes. As in the 60 Minutes story mentioned above, GAI-generated voicemails can trick an associate or family member into revealing passwords and other sensitive information to facilitate identity theft and a broad range of other fraudulent activities.
While AI-enabled identity hacks pose a significant security challenge, organizations do have options. One is liveness detection, an approach in which the user is required to go through a series of checks to prove that they are real and present at that moment. Examples include:
Passive facial liveness, which can recognize that a face is real without requiring movement
Active facial liveness, in which the user must perform specific actions in a predetermined order
Live voice recitation of a phrase
One-time passcodes or randomized PINs that the user must enter
In a report on remote identity proofing, ENISA recommends countering AI-enabled identity fraud through measures such as setting a minimum video quality level, checking user’s face depth to verify that it is three-dimensional and looking for image inconsistencies resulting from deepfake manipulation.
As cybersecurity advances in tandem with cyberthreats, the risks posed by AI can increasingly be countered using AI itself:
Authentication platforms incorporating AI technology can make it much easier to determine whether a scan of an individual’s biometric trait is valid and has been performed on a live individual in real time.
AI-powered identity and access management (AIM) systems can use behavioral analysis to establish a comprehensive profile of normal activity patterns for a specific user, including time, place and actions, and then flag any deviation from this norm to prompt additional authentication steps like multifactor or risk-based authentication.
Adaptive authentication technology can use machine learning (ML) to continuously assess user behavior and context to determine the associated level of risk. As the level of risk increases, more stringent and frequent authentication challenges can be posed.
Minimizing employee risks associated with AI
Public AI and ML systems have two key characteristics that increase their risk:
The AI/ML products and services have a public-facing portal as a direct offering to consumers, making them easily and widely available to anyone who wants to use them.
The registration of an account requires an individual’s explicit consent to non-negotiable legal terms that are heavily favorable to the AI/ML company—for example, allowing any information entered as part of a query to be used by the system for further training. In other words, if you input the transcript of a confidential strategy meeting to generate a summary, the contents of that meeting can now turn up verbatim in responses to future queries by the general public.
The use of public AI/ML systems by developers and security personnel can also create significant risks. The ability of ChatGPT to write and debug code has been hailed as a potential boon to developer productivity. In a recent GitHub survey, a full 92 percent of programmers indicated that they’re already using AI tools. But without an innate understanding of development concepts and contexts, these tools can easily introduce severe security vulnerabilities that are then introduced into the production environment by unwitting users.
On the security side, one survey found that 100 percent of SOC respondents want to use AI tools not provided by their company to work more easily, efficiently and/or effectively. Given the demands placed on these professionals by increasing speed, complexity and volume, this is entirely understandable, but highly concerning given the many known risks associated with shadow IT.
And as with meeting notes and other business content, the code entered into a query for debugging purposes can then be used to train the algorithm—and end up being incorporated into the code generated by the tool for other users.
To keep the GAI explosion from undermining security, CISOs need to move quickly and thoughtfully to implement policies governing use of AI tools across the enterprise. As a baseline, these rules should include:
Prohibiting the use of unsanctioned AI tools
Prohibiting employees and contractors from entering sensitive information such as code, meeting notes, intellectual property, and personal data into any AI query
Including language about the usage of AI tools in standard confidentiality agreements
Emerging best practices to govern employee use of AI tools include policies encompassing:
Data use. For example: Any company data must never be fed into a public-facing AI/ML system.
Quality assurance. For example: A human must always check the output from AI/ML systems to verify and validate its source and accuracy.
Data privacy. For example: Users must immediately delete any sensitive personal data received from the AI /ML system that is unrelated to their current activity.
Incident reporting. For example: If there has been an actual or suspected incident concerning the exposure of sensitive data to public facing AI/ML systems, it must be immediately reported to security personnel.
AI can be a powerful tool—but it can also create grave security issues. By taking a proactive, thoughtful and intentional approach to acceptable use policies within the company, and being vigilant about the potential threats arising outside, companies can ensure that the current wave of AI innovation doesn’t overwhelm their security posture.
Learn more about how AI can improve efficiency in the contract process.
Related posts
Discover what's new with Docusign IAM or start with eSignature for free
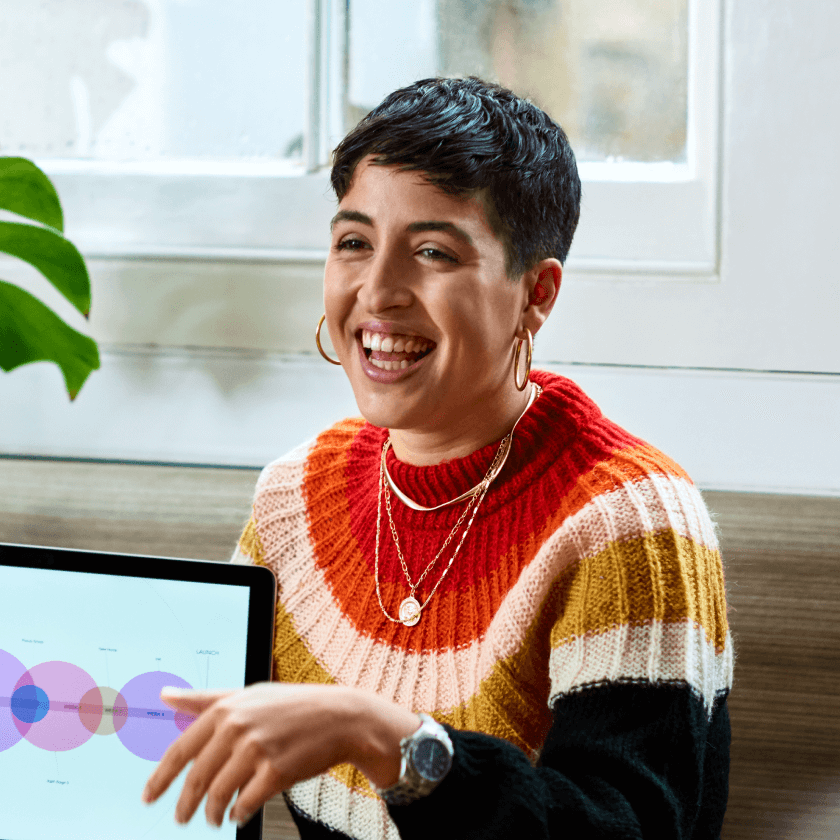